How should ad agencies measure the environmental cost of their AI use?
Awareness that generative AI carries an environmental cost is spreading, but how agencies might measure the impact of their usage is less clear.
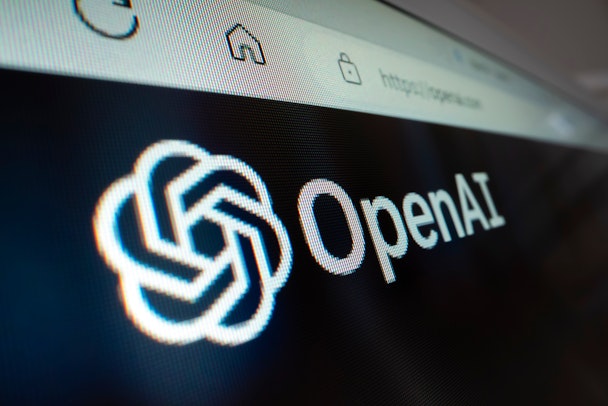
Creating tools such as ChatGPT consumes a lot of energy and water, implying a high environmental cost / Adobe Stock
Agency leaders are optimistic about business uses for generative AI, but they’re not considering the environmental impact of gen AI – even as it becomes clear the technology is thirsty for both electricity and water.
Over the course of the last year, several academic studies have identified generative AI as an energy-hungry technology. It takes a tremendous amount of electricity to power tools such as ChatGPT, with some estimates suggesting each query consumes as much energy as five regular search engine queries.
There are high energy costs involved in creating the large language models that GPT tools use, too. Researchers at Cornell University estimated that the creation of GPT-3 generated as much carbon emissions as 123 petrol-powered cars being driven for an entire year. In addition to energy usage, the cloud computing that powers most AI tools uses an enormous amount of water. The servers and computers – the physical infrastructure of AI – need to be kept cool to run effectively.
Worldwide water usage by Microsoft, for example, rose 34% between 2021 and 2022 – the equivalent of 2,500 Olympic-sized swimming pools – due mostly to its development of AI technology. According to researchers at the University of California, ChatGPT consumes 500ml of water every five to 50 queries, with this variation due to servers in different locations needing more or less coolant, as well as estimates of water usage at power plants supplying servers with electricity.
Explore frequently asked questions
Most advertising agencies are experimenting and piloting applications of gen AI right now, most of which rest upon either using existing generative AI tools with premade LLMs or building bespoke underlying models for clients. They’re not, however, measuring the environmental costs of those experiments or considering what the environmental costs might be.
According to AI and sustainability experts, the methodology for calculating the carbon and water costs of the tech is already with us, but agencies need to consider the size of the models they’re working with, the complexity and diversity of tools out there and then begin buttonholing AI partners for more information.
Methods already exist, but the data doesn’t
George Strakhov, chief strategist of DDB’s European business and the founder of its dedicated AI business, Rand DDB, says the company has access to measurement frameworks for the environmental impact of AI because of its links to OPMG, an Omnicom media agency. DDB’s parent is “very diligent” in tracking its environmental impact, he claims, but adds that it lacks the “granularity” to apply those methodologies to AI usage in its projects.
“I don’t think we’re there yet,” he says, partly because Rand’s actual use of AI has stayed small scale. “What we’re focused on is ‘atomic’ uses, experiments and individual generations for clients in different situations. We are working towards making sure that those are trackable, but the reality is they’re relatively tiny.”
For agencies that want to try and account for the environmental costs of AI, there are frameworks that can be used – for example, Scope 2, the industry-wide standard for measuring carbon emissions related to a business’ supply chain. It would apply to agencies using ChatGPT or Stable Diffusion, just as it would other software.
According to Rachel Delacour, chief executive of Sweep, a company that maps business activities against their direct and indirect carbon and water costs, most businesses already have the relevant data to hand, even if they haven’t yet translated it into its cost in carbon emissions. “Granularity means auditability,” she says.
Waste and water
Though it’s not what most people think about when they consider environmental costs, Delacour says it’s possible to track water usage using the same methodology used for carbon. In fact, she says it’s easier.
“In the ESG [environmental, social, and corporate governance] field, the ‘E’ is the most complex part, and within ‘E,’ carbon is the most difficult.” By comparison, calculating water usage is relatively straightforward. “We’ve tackled the north face.”
Chris Neff, global head of emerging experience and technology at Stagwell-owned agency Anomaly, says it’s “difficult to quantify” the environmental impact of AI usage or large language model training. “There’s a massive difference between GPT-4 versus Bard versus Llama,” he notes.
Advertisement
Different types of tools use more electricity and likely more water, he says. Runway – the generative video tech recently used by Publicis Groupe to create 100,000 personalized films for staff – can take up to half a minute to render an asset. “What’s going on in those segments implies a lot of computing power,” says Neff.
That might not remain a major issue. Bespoke LLMs or image-based training datasets – the creation of which might become a major agency service in years to come, according to Forrester – are much smaller than the ones behind ChatGPT. “The processing power is far, far smaller,” says Neff. “You might not even need the cloud; you might be able to use it locally.
“If we have our own box, our own fine-tuned models, we don’t have to deal with the inefficiencies of the bigger models and can be more calculated and mindful of our access, our use and our footprint.”
The size of a given model isn’t the only important variable worth thinking about. Where the power is generated also matters. For example, the open-source Bloom LLM, which was created by startup Hugging Face, was trained on a French supercomputer using electricity from the primarily nuclear-powered French electricity grid, meaning there were far fewer carbon emissions generated than if it had been built elsewhere. Delacour notes that methodologies already exist to take account of such nuance.
Ryan Cochrane, the chief operating officer of sustainable programmatic firm Good-Loop, says similar effects have been long observed in the media buying space. “There can be huge differences in ad serving around the time of day and the country in which you’re doing it. The electricity grid in Australia, for example, is super coal-powered, but if you set up server farms in Ontario, which uses hydroelectric power, your CO2 usage is pretty minimal.”
He points out that by working with open-source technologies such as Bloom, private companies can save energy and water. “We see more emissions from the development and training of models, and we’re seeing huge amounts of duplication, but there are a lot of open-source projects that can be tapped into and used. You don’t want to train a whole language model, with the environmental footprint that comes with that, if the work is already there and available.”
Advertisement
Good-Loop measures the environmental impact of media and creative activity but is not yet measuring the impact of gen AI. “It’s not something we currently factor into our methodology,” says Cochrane. There are, however, projects emerging to take on the topic, he adds, such as the Green AI Standard.
The biggest obstacle, though, is a lack of transparency from market leaders in the AI space. Despite its non-profit corporate structure, OpenAI, for example, hasn’t disclosed detailed information on its energy or water usage, with academic studies so far based on estimates.
Without a comprehensive perspective on the actual energy and water use behind tools such as ChatGPT or Dall-E, agencies will be unable to generate accurate estimates relating to their own use. “It’s good to start with estimates, I would say, but it’s absolutely not enough,” says Delacour.
Cochrane adds: “There is a real lack of documentation around how these models are being assessed and, without that, you can’t reliably assign a carbon score.”
That could change, of course. Most of the industry’s big players have established formal links or partnerships with AI software publishers such as Adobe and OpenAI. They could use those relationships to ask for more information on the environmental costs of the tech.
“First, this is a matter of understanding what it will cost and optimizing your use,” says Delacour. ”Second, it’s about asking questions about the footprint. At the core of this is the willingness of providers to do the work of understanding [the impact] and then inform their customers. It is on us, on users, to ask for this and not wait for years like we’ve been waiting on cloud services.”
Suggested newsletters for you
In an email after our interview, Strakhov says Rand is already taking environmental impacts into account in choosing those partners: ”We fully support the efforts to minimize direct climate impacts of AI and computation and data explosion in general. We work with partners and providers who do this to the best of currently possible standards.”
He argues that any accounting should weigh up the potential environmental benefits unlocked by AI. Virtual production, he suggests, could replace flying out staff for location shoots – especially if it’s made more efficient or more powerful by incorporating AI. Cochrane says that he and others hope AI can reach a “tipping point” at which it becomes effective enough to provoke that change.
Ideally, any accounting of the impact of AI would include the ’S’ in ESG, with Strakhov explaining: “A big part of the potential downside of AI is societal impact – impact on human psychology, impact on culture – and this is something that we can’t quantify, but something that we try our best to be very mindful of and think carefully about the impacts here.“
In the shorter term, Delacour argues, AI companies themselves have a social duty to disclose more data. “The big names of AI, the Googles and AWSs and Microsofts, will have to open their books. We have to have the right data from providers. You can benchmark, you can assume, but in today’s world, it is the responsibility of everyone who is in the business of making anything to account for their carbon emissions.”